Machine Learning and Electronic Clinical Decision Support Systems for or Against Individualized and Precision Approach to the Newborn Diseases
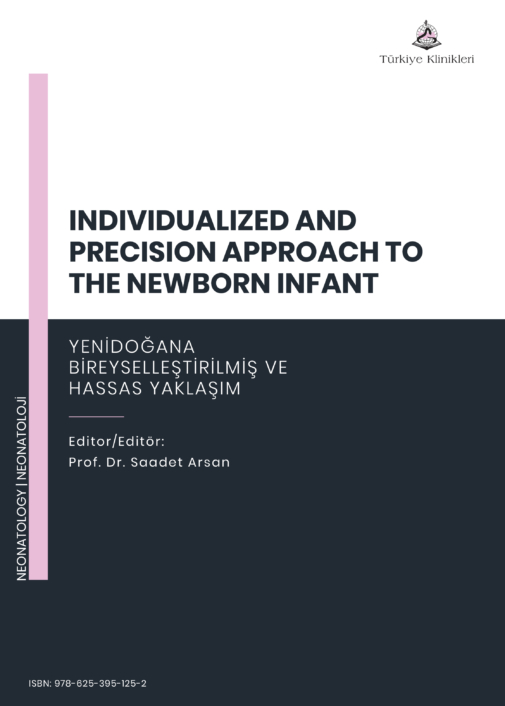
Sara EROLa
aAnkara Yıldırım Beyazit University Faculty of Medicine, Department of Pediatrics, Division of Neonatology, Ankara, Türkiye
ABSTRACT
The utilization of machine learning and electronic decision support systems in neonatal care is increasing in parallel with the growing impact of artificial intelligence in the healthcare. These systems analyse genetic, environmental, and lifestyle data to achieve and promote individualized and precision approach, which is indispensable for the most vulnerable population: the newborns. As novel tools are being developed to provide critical solutions in various areas for newborns and their families, clinical care team members, researchers, community health program managers, and healthcare business administrators, the possibility of encountering unintended results and the presence of ethical concerns will be important topics of discussion.
Keywords: Precision medicine; machine learning; newborn
Citation
Referanslar
- World Health Organization. Newborn deaths [Internet] (cited: 2023 August 25) Available online at: [Link]
- World Health Organization. Every Newborn: an action plan to end preventable deaths. [Internet] (cited: 2023 August 25) Available online at: [Link]
- Lawn JE, Blencowe H, Oza S, You D, Lee AC, Waiswa P, et al; Lancet Every Newborn Study Group. Every Newborn: progress, priorities, and potential beyond survival. Lancet. 2014;384(9938):189-205. Erratum in: Lancet. 2014; 384(9938):132. [Crossref] [PubMed]
- Lawn JE, Ohuma EO, Bradley E, Idueta LS, Hazel E, Okwaraji YB, et al; Lancet Small Vulnerable Newborn Steering Committee; WHO/UNICEF Preterm Birth Estimates Group; National Vulnerable Newborn Measurement Group; Subnational Vulnerable Newborn Measurement Group. Small babies, big risks: global estimates of prevalence and mortality for vulnerable newborns to accelerate change and improve counting. Lancet. 2023; 401(10389):1707-19. [Crossref] [PubMed]
- National Human Genome Research Institute. Precision Medicine. [Internet] (cited: 2023 August 25) Available online at: [Link]
- National Human Genome Research Institute. Personalized Medicine. [Internet] (cited: 2023 August 25) Available online at: [Link]
- National Library of Medicine. What is the difference between precision medicine and personalized medicine? What about pharmacogenomics? (cited: 2023 August 25) Available online at: [Link]
- Delpierre C, Lefèvre T. Precision and personalized medicine: What their current definition says and silences about the model of health they promote. Implication for the development of personalized health. Front Sociol. 2023; 8:1112159. [Crossref] [PubMed] [PMC]
- Johnson KB, Wei WQ, Weeraratne D, Frisse ME, Misulis K, Rhee K, et al. Precision Medicine, AI, and the Future of Personalized Health Care. Clin Transl Sci. 2021;14(1):86-93. [Crossref] [PubMed] [PMC]
- Kwok TC, Henry C, Saffaran S, Meeus M, Bates D, Van Laere D, et al. Application and potential of artificial intelligence in neonatal medicine. Semin Fetal Neonatal Med. 2022;27(5):101346. [Crossref] [PubMed]
- MacEachern SJ, Forkert ND. Machine learning for precision medicine. Genome. 2021;64(4):416-25. [Crossref] [PubMed]
- Rao A, Palma J. Clinical decision support in the neonatal ICU. Semin Fetal Neonatal Med. 2022;27(5):101332. [Crossref] [PubMed]
- Matheny ME, Whicher D, Thadaney Israni S. Artificial Intelligence in Health Care: A Report From the National Academy of Medicine. JAMA. 2020;323(6):509-10. [Crossref] [PubMed]
- Liang YC, Wijaya I, Yang MT, Cuevas Juarez JR, Chang HT. Deep Learning for Infant Cry Recognition. Int J Environ Res Public Health. 2022;19(10):6311. [Crossref] [PubMed] [PMC]
- Revathi KP, Manikandan T. A Smart and Secured Approach for Children's Health Monitoring Using Machine Learning Techniques Enhancing Data Privacy. IETE Journal of Research. 2023;69(3):1210-21. [Crossref]
- Jiménez-Serrano S, Tortajada S, García-Gómez JM. A Mobile Health Application to Predict Postpartum Depression Based on Machine Learning. Telemed J E Health. 2015;21(7):567-74. [Crossref] [PubMed]
- Chua JYX, Choolani M, Chee CYI, Yi H, Chan YH, Lalor JG,et al. 'Parentbot - A Digital healthcare Assistant (PDA)': A mobile application-based perinatal intervention for parents: Development study. Patient Educ Couns. 2023; 114:107805. [Crossref] [PubMed]
- McAdams RM, Kaur R, Sun Y, Bindra H, Cho SJ, Singh H. Predicting clinical outcomes using artificial intelligence and machine learning in neonatal intensive care units: a systematic review. J Perinatol. 2022;42(12):1561-75. [Crossref] [PubMed]
- Mangold C, Zoretic S, Thallapureddy K, Moreira A, Chorath K, Moreira A. Machine Learning Models for Predicting Neonatal Mortality: A Systematic Review. Neonatology. 2021;118(4):394-405. [Crossref] [PubMed] [PMC]
- Sharifi-Heris Z, Laitala J, Airola A, Rahmani AM, Bender M. Machine Learning Approach for Preterm Birth Prediction Using Health Records: Systematic Review. JMIR Med Inform. 2022;10(4):e33875. [Crossref] [PubMed] [PMC]
- Sullivan BA, Kausch SL, Fairchild KD. Artificial and human intelligence for early identification of neonatal sepsis. Pediatr Res. 2023;93(2):350-6. [Crossref] [PubMed]
- López-Martínez F, Núñez-Valdez ER, Gomez JL, García-Díaz V. A neural network approach to predict early neonatal sepsis. Computers & Electrical Engineering, 2019;76:379-88. [Crossref]
- Darsareh F, Ranjbar A, Farashah MV, Mehrnoush V, Shekari M, Jahromi MS. Application of machine learning to identify risk factors of birth asphyxia. BMC Pregnancy Childbirth. 2023;23(1):156. [Crossref] [PubMed] [PMC]
- Chinnaiyan R, Alex S. Machine Learning Approaches for Early Diagnosis and Prediction of Fetal Abnormalities. In: 2021 International Conference on Computer Communication and Informatics Coimbatore. India: 2021. p. 1-3. [Crossref]
- Daunhawer I, Kasser S, Koch G, Sieber L, Cakal H, Tütsch J, et al. Enhanced early prediction of clinically relevant neonatal hyperbilirubinemia with machine learning. Pediatr Res. 2019;86(1):122-7. [Crossref] [PubMed]
- Ahmed W, Veluthandath AV, Rowe DJ, Madsen J, Clark HW, Postle AD, et al. Prediction of Neonatal Respiratory Distress Biomarker Concentration by Application of Machine Learning to Mid-Infrared Spectra. Sensors (Basel). 2022; 22(5):1744. [Crossref] [PubMed] [PMC]
- Natarajan A, Lam G, Liu J, Beam AL, Beam KS, Levin JC. Prediction of extubation failure among low birthweight neonates using machine learning. J Perinatol. 2023;43(2):209-14. [Crossref] [PubMed] [PMC]
- Dai D, Chen H, Dong X, Chen J, Mei M, Lu Y, et al. Bronchopulmonary Dysplasia Predicted by Developing a Machine Learning Model of Genetic and Clinical Information. Front Genet. 2021;12:689071. [Crossref] [PubMed] [PMC]
- Na JY, Kim D, Kwon AM, Jeon JY, Kim H, Kim CR, et al. Artificial intelligence model comparison for risk factor analysis of patent ductus arteriosus in nationwide very low birth weight infants cohort. Sci Rep. 2021;11(1):22353. [Crossref] [PubMed] [PMC]
- Smyser CD, Dosenbach NU, Smyser TA, Snyder AZ, Rogers CE, Inder TE, et al. Prediction of brain maturity in infants using machine-learning algorithms. Neuroimage. 2016;136:1-9. [Crossref] [PubMed] [PMC]
- Ihlen EAF, Støen R, Boswell L, Regnier RA, Fjørtoft T, Gaebler-Spira D, et al. Machine Learning of Infant Spontaneous Movements for the Early Prediction of Cerebral Palsy: A Multi-Site Cohort Study. J Clin Med. 2019;9(1):5. [Crossref] [PubMed] [PMC]
- Demirci GM, Kittler PM, Phan HTT, Gordon AD, Flory MJ, Parab SM, Tsai CL. Predicting mental and psychomotor delay in very pre-term infants using machine learning. Pediatr Res. 2023 Jul 27. [Crossref] [PubMed] [PMC]
- Valavani E, Blesa M, Galdi P, Sullivan G, Dean B, Cruickshank H, et al. Language function following preterm birth: prediction using machine learning. Pediatr Res. 2022;92(2):480-9. [Crossref] [PubMed] [PMC]
- Pavel AM, Rennie JM, de Vries LS, Blennow M, Foran A, Shah DK, et al. A machine-learning algorithm for neonatal seizure recognition: a multicentre, randomised, controlled trial. Lancet Child Adolesc Health. 2020;4(10):740-9. [Crossref] [PubMed] [PMC]
- Liu Z, Ji B, Zhang Y, Cui G, Liu L, Man S, Ding L, Yang X, Mao H, Wang L. Machine Learning Assisted MRI Characterization for Diagnosis of Neonatal Acute Bilirubin Encephalopathy. Front Neurol. 2019;10:1018. [Crossref] [PubMed] [PMC]
- Abbasi H, Unsworth CP. Applications of advanced signal processing and machine learning in the neonatal hypoxic-ischemic electroencephalogram. Neural Regen Res. 2020;15(2):222-31. [Crossref] [PubMed] [PMC]
- Attallah O. DIAROP: Automated Deep Learning-Based Diagnostic Tool for Retinopathy of Prematurity. Diagnostics (Basel). 2021;11(11):2034. [Crossref] [PubMed] [PMC]
- McKearney RM, MacKinnon RC. Objective auditory brainstem response classification using machine learning. Int J Audiol. 2019;58(4):224-30. [Crossref] [PubMed]
- Honoré A, Forsberg D, Adolphson K, Chatterjee S, Jost K, Herlenius E. Vital sign-based detection of sepsis in neonates using machine learning. Acta Paediatr. 2023;112(4):686-96. [Crossref] [PubMed]
- Althnian A, Almanea N, Aloboud N. Neonatal Jaundice Diagnosis Using a Smartphone Camera Based on Eye, Skin, and Fused Features with Transfer Learning. Sensors (Basel). 2021;21(21):7038. [Crossref] [PubMed] [PMC]
- Young BK, Cole ED, Shah PK, Ostmo S, Subramaniam P, Venkatapathy N, et al. Efficacy of Smartphone-Based Telescreening for Retinopathy of Prematurity With and Without Artificial Intelligence in India. JAMA Ophthalmol. 2023;141(6):582-8. [Crossref] [PubMed] [PMC]
- Hu Y, Gong X, Shu L, Zeng X, Duan H, Luo Q, et al. Understanding risk factors for postoperative mortality in neonates based on explainable machine learning technology. J Pediatr Surg. 2021;56(12):2165-71. [Crossref] [PubMed]
- McElroy SJ, Lueschow SR. State of the art review on machine learning and artificial intelligence in the study of neonatal necrotizing enterocolitis. Front Pediatr. 2023;11:1182597. [Crossref] [PubMed] [PMC]
- Nakamura H, Yamataka A. Non-invasive and accurate diagnostic system for biliary atresia. EBioMedicine. 2018;36:16-17. [Crossref] [PubMed] [PMC]
- Huang SG, Qian XS, Cheng Y, Guo WL, Zhou ZY, Dai YK. Machine learning-based quantitative analysis of barium enema and clinical features for early diagnosis of short-segment Hirschsprung disease in neonate. J Pediatr Surg. 2021;56(10):1711-7. [Crossref] [PubMed]
- Lure AC, Du X, Black EW, Irons R, Lemas DJ, Taylor JA, et al. Using machine learning analysis to assist in differentiating between necrotizing enterocolitis and spontaneous intestinal perforation: A novel predictive analytic tool. J Pediatr Surg. 2021;56(10):1703-10. [Crossref] [PubMed]
- Van Laere D, Meeus M, Beirnaert C, Sonck V, Laukens K, Mahieu L, et al. Machine Learning to Support Hemodynamic Intervention in the Neonatal Intensive Care Unit. Clin Perinatol. 2020;47(3):435-48. [Crossref] [PubMed]
- O'Sullivan C, Tsai DH, Wu IC, Boselli E, Hughes C, Padmanabhan D, et al. Machine learning applications on neonatal sepsis treatment: a scoping review. BMC Infect Dis. 2023;23(1):441. [Crossref] [PubMed] [PMC]
- Ponthier L, Ensuque P, Destere A, Marquet P, Labriffe M, Jacqz-Aigrain E, et al.Optimization of Vancomycin Initial Dose in Term and Preterm Neonates by Machine Learning. Pharm Res. 2022;39(10):2497-506. [Crossref] [PubMed]
- Shirwaikar RD. Estimation of Caffeine Regimens: A Machine Learning Approach for Enhanced Clinical Decision Making at a Neonatal Intensive Care Unit (NICU). Crit Rev Biomed Eng. 2018;46(2):93-115. [Crossref] [PubMed]
- do Nascimento ARF, Greati VR, Leopoldino RWD, Martins RR, Barbosa EG. Machine Learning for Prediction of Adverse Drug Reactions: Application to Neonatal Intensive Care Units. Available at SSRN: https://ssrn.com/abstract=4462705 or http://dx.doi.org/10.2139/ssrn.4462705 [Crossref]
- Vinks AA, Punt NC, Menke F, Kirkendall E, Butler D, Duggan TJ, et al. Electronic Health Record-Embedded Decision Support Platform for Morphine Precision Dosing in Neonates. Clin Pharmacol Ther. 2020;107(1):186-94. [Crossref] [PubMed] [PMC]
- Zaunseder E, Haupt S, Mütze U, Garbade SF, Kölker S, Heuveline V. Opportunities and challenges in machine learning-based newborn screening-A systematic literature review. JIMD Rep. 2022;63(3):250-61. [Crossref] [PubMed] [PMC]
- Usha Rani G, Kadali S, Kurma Reddy B, Shaheena D, Naushad SM. Application of machine learning tools and integrated OMICS for screening and diagnosis of inborn errors of metabolism. Metabolomics. 2023;19(5):49. [Crossref] [PubMed]
- Porras AR, Rosenbaum K, Tor-Diez C, Summar M, Linguraru MG. Development and evaluation of a machine learning-based point-of-care screening tool for genetic syndromes in children: a multinational retrospective study. Lancet Digit Health. 2021;3(10):e635-e43. [Crossref] [PubMed]
- Bucher SL, Rajapuri A, Ravindran R, Rukunga J, Horan K, Esamai F, et al. The Essential Care For Every Baby Digital Action Plan: Design And Usability Testing Of A Mobile Phone-Based Newborn Care Decision Support Tool In Kenya. AAP National Conference & Exhibition Meeting Abstracts 2021; 147(3):263-4. [Crossref]
- P Fishler K, Euteneuer JC, Brunelli L. Ethical Considerations for Equitable Access to Genomic Sequencing for Critically Ill Neonates in the United States. Int J Neonatal Screen. 2022 Mar 21;8(1):22. [Crossref] [PubMed] [PMC]
- Dorn SD. Digital Health: Hope, Hype, and Amara's Law. Gastroenterology. 2015;149(3):516-20. [Crossref] [PubMed]
- Scott IA. Demystifying machine learning: a primer for physicians. Intern Med J. 2021;51(9):1388-400. [Crossref] [PubMed]
- Cabitza F, Rasoini R, Gensini GF. Unintended Consequences of Machine Learning in Medicine. JAMA. 2017;318(6):517-8. [Crossref] [PubMed]
- d'Elia A, Gabbay M, Rodgers S, Kierans C, Jones E, Durrani I, et al. Artificial intelligence and health inequities in primary care: a systematic scoping review and framework. Fam Med Community Health. 2022;10(Suppl 1):e001670. [Crossref] [PubMed] [PMC]
- Woerner AC, Gallagher RC, Vockley J, Adhikari AN. The Use of Whole Genome and Exome Sequencing for Newborn Screening: Challenges and Opportunities for Population Health. Front Pediatr. 2021;9:663752. [Crossref] [PubMed] [PMC]
- Friedman JM, Cornel MC, Goldenberg AJ, Lister KJ, Sénécal K, Vears DF; Global Alliance for Genomics and Health Regulatory and Ethics Working Group Paediatric Task Team. Genomic newborn screening: public health policy considerations and recommendations. BMC Med Genomics. 2017;10(1):9. [Crossref] [PubMed] [PMC]
- Fost N. Informed Consent Should Be a Required Element for Newborn Screening, Even for Disorders with High Benefit-Risk Ratios. J Law Med Ethics. 2016;44(2):241-55. [Crossref] [PubMed]
- Michelson KN, Klugman CM, Kho AN, Gerke S. Ethical Considerations Related to Using Machine Learning-Based Prediction of Mortality in the Pediatric Intensive Care Unit. J Pediatr. 2022;247:125-8. [Crossref] [PubMed] [PMC]
- Achampong EK, Adzakpah G, Boadu RO, Lasim O. The Quality of Newborn Data: Assessment of Data Management and Reporting System. Int J Public Heal Sci. 2018;7:194-200. [Crossref]
- Dicuonzo G, Donofrio F, Fusco A, Shini M. Healthcare system: Moving forward with artificial intelligence. Technovation. 2023;120:102510. [Crossref]
- U.S. Food and Drug Administration. Artificial Intelligence and Machine Learning (AI/ML)-Enabled Medical Devices. [Internet] (cited: 2023 August 25) Available online at: [Link]
- U.S. Government Accountability Office, Artificial Intelligence in Health Care: Benefits and Challenges of Machine Learning in Drug Development. [Internet] (cited: 2023 August 25) Available online at: [Link]
- Arora A. Moravec's paradox and the fear of job automation in health care. Lancet. 2023;402(10397):180-1. [Crossref] [PubMed]